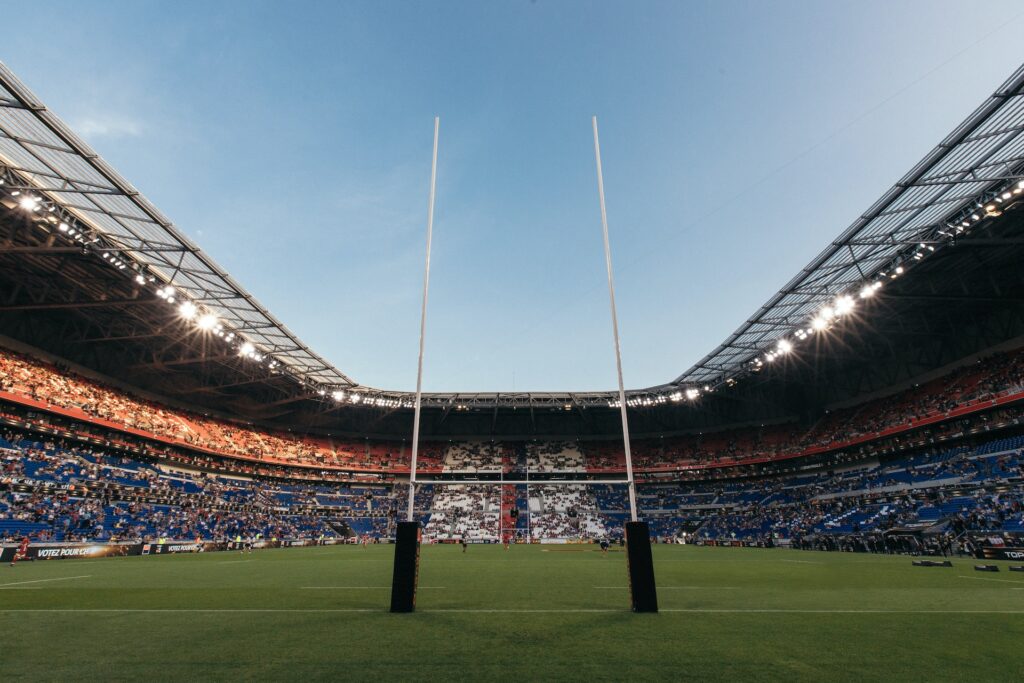
Introduction
The exciting world of sports betting merges with the exacting science of statistics to unlock novel strategies for th ose engaging in the art of making profitable Ligue 1 bets. Amid the thrilling twists and turns of French football, savvy bettors are increasingly turning to predictive analytics for football as a means of gaining a competitive edge. In this realm, where every statistical angle and historical trend is dissected, the construction of sophisticated models is paramount.
As we investigate the intricacies of these predictive endeavors, it becomes clear that the development of successful sports betting strategies hinges upon a deep comprehension of past performances, probability calculations, and the subtle nuances that define Ligue 1 competition. The careful analysis and adaptation of statistical methodologies pave the way for sharper insights and, consequently, more informed betting decisions.
Understanding the Foundations of Football Statistical Models
The development of statistical analysis techniques has revolutionized the way we predict and interpret football matches. Taking into account the complexities inherent in the sport, statistical models provide a framework for generating football match predictions that are more sophisticated than simple guesswork. The robust framework of these models springs from the work of pioneers like Maher, whose 1982 model laid the groundwork for today’s advanced modeling techniques for sports forecasting.
Central to Maher’s proposition was the usage of a Poisson distribution to project the attacking and defensive capabilities of teams, which inherently included home-field advantage as a significant determinant. This concept was critical in constructing the foundation of our current understanding of team performance patterns. Nevertheless, the volatility in football demanded more than just fixed coefficients; it required a flexible system that could account for the variability and unpredictability of match outcomes.
Emerging from this need were Bayesian methodologies, updating the model’s assumptions to better align with real-world football dynamics. These methodologies introduced the concept of utilizing random variables to mirror team abilities—an advancement that more closely mirrored the outcomes seen on the pitch. This strategic application of Bayesian inferences enabled the models to account for over-dispersion in the number of goals scored, providing a more accurate fit for match results than the original Poisson calculation could offer.
Progressively, it has become clear that the sophisticated art of statistical modeling is not static; it is in perpetual development, being refined to increase its forecasting precision. This continuous evolution in modeling techniques for sports forecasting is not just academic; it plays a consequential role in the ongoing battle against bookmakers, ensuring that the insights gained facilitate a deeper and more nuanced understanding of the nature of football contests.
Assessing Team Strengths and Weaknesses in Ligue 1 Betting
In the meticulous world of sports betting, Ligue 1 stands as a vibrant arena where understanding team performance nuances can provide a significant betting edge. The precision in predictive model accuracy hinges on a comprehensive analysis, dissecting the multifaceted layers of team capabilities. Particularly vital is deciphering both attacking and defensive proficiencies, as these metrics lay the groundwork for anticipating the possible number of goals teams may score or concede.
Historical data patterns are instrumental in Ligue 1 team performance analysis. Dixon and Coles introduced a pioneering approach by incorporating a time-dependent factor that accounts for temporary yet impactful variables, such as fluctuating player rosters and managerial tactics. This intricate understanding of changes within a team’s anatomy and their possible effect on upcoming matches is central to maintaining a model’s predictive accuracy amidst the ebb and flow of a dynamic football league.
Let’s examine a comparison of Ligue 1 teams’ offensive and defensive ratings which directly influence betting models:
Team | Offensive Rating | Defensive Rating | Recent Form Indicator |
---|---|---|---|
Paris Saint-Germain | 2.35 | 0.85 | Stable |
Olympique Lyonnais | 1.75 | 1.20 | Improving |
Lille OSC | 1.50 | 1.10 | Declining |
Olympique de Marseille | 1.65 | 0.95 | Unstable |
AS Monaco | 1.80 | 1.25 | Stable |
This table not only distinguishes each team’s potential but also reveals fluctuations that could redefine betting strategies. Recognizing these variances as more than statistical outliers but as reflections of evolving strategies and player performance can give bettors an informed advantage. Successful betting in Ligue 1 doesn’t just depend on raw data; it’s about the analytical acumen to translate those numbers into actionable insights.
Advanced Metrics and Their Impact on Football Model Accuracy
The integration of Ligue 1 advanced metrics into predictive modeling is a game-changer for football data analytics. Today’s sports analysts have a wealth of in-game stats at their disposal, ranging from passes completed to the distance covered by each player. This granular data offers a more nuanced view of a team’s performance, thereby increasing the precision of accurate betting predictions. As the table below illustrates, these innovative metrics offer insights that traditional stats simply cannot provide.
Traditional Metric | Advanced Metric | Insight Gained |
---|---|---|
Goals Scored | Expected Goals (xG) | Measures the quality of a shot based on several variables, offering a more accurate prediction of scoring chances. |
Shots on Goal | Shots on Target Percentage | Provides context to the likelihood of a shot leading to a goal, factoring in accuracy beyond just volume. |
Goalkeeper Saves | Post-Shot Expected Goals – Goals Allowed (PSxG-GA) | Assesses a goalkeeper’s performance by comparing expected goals on target against actual goals conceded. |
Pass Completion Rate | Progressive Passes | Tracks the forward movements leading to attacking opportunities, thus evaluating the impact of a player’s distribution. |
Ball Possession | Under Pressure Actions | Examines team’s and players’ composure and decision-making skills when facing defensive aggression. |
By embracing the detailed data that modern football analysis affords, sports bettors and enthusiasts alike can delve into the intricacies of the game. This deeper understanding cultivates an environment where Ligue 1 advanced metrics hold sway in the complex yet captivating world of football betting.
Implementing Bayesian Models for Enhanced Ligue 1 Predictions
The practice of Bayesian inference in betting has transformed the landscape of predictive models for football. Bettors now consider this advanced statistical methodology a cornerstone of data-driven betting strategies, devised to sharpen the accuracy of Ligue 1 match predictions. The appeal of Bayesian models lies in their capacity to incorporate prior knowledge and adjust to new data, emphasizing an iterative process that aligns closer with the dynamic nature of the sport.
Model Component | Description | Advantages |
---|---|---|
Prior Probability Distribution | Integrates existing data about team performances. | Allows the model to start from a historically informed baseline. |
Likelihood Function | Assesses the probability of observed data given model parameters. | Facilitates model adjustment based on the latest game outcomes. |
Posterior Probability | Updates the model with new evidence to refine future predictions. | Optimizes predictions through continual learning from recent matches. |
Over-dispersion | Accounts for variance in goals outside Poisson distribution expectations. | Enhances model realism by recognizing the unpredictability inherent in football. |
The implementation of Bayesian models not only improves the pinpointing of a team’s current strengths and vulnerabilities but also lends itself to a more nuanced appreciation of the uncertainties that permeate football betting. As a quantitative framework, Bayesian inference harmonizes the ostensibly opposing tasks of maintaining model rigor while accommodating the sport’s inherent volatility. Through integrating complex probability distributions and historical match data, Bayesian models invariably mature into formidable tools for forecasting the intensely contested matches of Ligue 1.
Dynamic Models: Capturing the Fluctuations in Ligue 1 Team Performances
The forefront of statistical innovation in sports betting is now dominated by dynamic betting models, which adeptly track team performance trends. Through the lens of predictive analysis in sports betting, these models are instrumental in distilling the essence of a team’s performance over the course of a season. One of the pivotal systems that illustrate the potency of fluctuations in sports analytics is the model developed by Rue and Salvesen. It employs a sophisticated random-walk approach to account for inherent changes in team abilities—modifying the predictions based on the ‘memory’ of past performance statistics.
The uninterrupted advancements in dynamic betting models are pivotal in parsing out the nuances of predictive analysis in sports betting. As bettors and sports analysts seek to refine their strategies, it becomes clear that understanding the trends is vital for informed betting decisions. To exemplify the critical variables processed by these dynamic betting models, a comparative table of fluctuating team performance metrics in Ligue 1 offers an insightful glance into the complexity and utility of these predictive tools.
Season | Team | Attacking Strength | Defensive Strength | Form Variation |
---|---|---|---|---|
2020/21 | Paris Saint-Germain | 2.3 | 0.8 | Stable |
2021/22 | Olympique Lyonnais | 2.0 | 1.2 | Improving |
2022/23 | Lille OSC | 1.8 | 1.1 | Declining |
2023/24 | AS Monaco | 2.1 | 0.9 | Unstable |
In dissecting the table above, it is evident that a team’s performance in Ligue 1 is subject to fluctuation, much like the broader stock market trends. Dynamic betting models make it possible to scrutinize these trends and adapt betting strategies in real-time. The outcome is a more precise and tailored approach to sports betting, where each wager is supported by a deep well of analytical data, responding to the ever-changing tableau of team performances.
Integrating External Data for Superior Ligue 1 Betting Predictions
The sphere of Ligue 1 betting goes well beyond in-match statistics and historical performance data. For those seeking to refine their betting edge, Ligue 1 betting data integration that incorporates external datasets becomes an important strategy. Broadening the analytical viewfinder to include factors like playoff dynamics, team hierarchy, and variances in seasonal performance patterns ensures a more nuanced prediction model. Performance tracking becomes not only about past scores and in-game execution but also about understanding how teams respond to league structures and competitive pressures over the course of a season.
Recognizing the significance of external data for betting is essential to improving the sophistication of forecasting methods. Whether it’s accounting for unexpected events such as weather disruption, considering the psychological impacts of rivalry matches, or analyzing transfer market movements, each element plays a critical role in shaping game outcomes. This multiplicity of factors, when meticulously blended with traditional prediction models, can heighten the accuracy of anticipations, thereby providing punters with a considerable advantage against standard betting practices.
Leveraging the full gamut of sporting analytics available reflects a deep comprehension of the dynamics at play within Ligue 1. It is this depth of knowledge and the agility to integrate disparate data points that lift predictive modeling to a higher echelon of precision. As punters and analysts alike seek superior strategies, the role of external data is not only to inform but to transform betting approaches, ensuring that every bit of accessible information is harnessed to construct the most robust betting predictions possible.
FAQ
How do statistical models enhance predictive analytics for football betting?
Statistical models leverage historical game data and apply mathematical techniques to forecast future game outcomes. They incorporate various factors like team strengths, game strategies, and player performance, providing a quantitative basis for making profitable Ligue 1 bets and forming sports betting strategies.
What are the benefits of using predictive models for Ligue 1 betting?
Using predictive models for Ligue 1 betting allows bettors to make more informed decisions by analyzing team trends and patterns. This can lead to a higher success rate in betting and potentially provide a more systematic and analytic approach to gambling.
What is the importance of statistical analysis in football match predictions?
Statistical analysis is critical in extracting meaningful patterns and trends from past football matches. This information forms the foundation of predictive models aiming to forecast future match outcomes. It aids in quantifying the strengths and weaknesses of teams, ultimately improving modeling techniques for sports forecasting.
How have modeling techniques for sports forecasting evolved over time?
Modeling techniques for sports forecasting have advanced from simple probabilistic models to complex systems that incorporate machine learning and AI. These models now factor in dynamic variables, provide real-time analytics, and adapt to new data, offering a more sophisticated approach to predicting match outcomes.
Why is understanding Ligue 1 team performance analysis crucial for betting?
Understanding team performance in Ligue 1 allows bettors to assess which teams have a statistical edge in upcoming matches. This analysis helps in predicting outcomes more accurately, factoring in elements like current form, historical performances, and head-to-head statistics.
How does a predictive model determine the accuracy of Ligue 1 betting?
Predictive models determine the accuracy of betting by how well they can forecast the outcomes of matches compared to actual results. They use various performance metrics and statistical tools to evaluate the probability of different scenarios, enhancing the betting edge over bookmakers.
What role do advanced metrics play in improving football model accuracy?
Advanced metrics offer deeper insights into a team’s playing conditions and the effectiveness of their playing style, providing a more nuanced view of expected performance. They include data points like possession rates, player movements, and detailed shot analytics, which help sharpen the accuracy of betting predictions.
How have advanced metrics changed the approach to Ligue 1 betting predictions?
Advanced metrics have provided bettors and analysts with finer details beyond basic game stats, such as goals scored and conceded. This high-resolution data supports more nuanced statistical models that can better predict game outcomes and identify value bets.
What advantages do Bayesian models offer in the context of Ligue 1 betting predictions?
Bayesian models incorporate prior knowledge and continually update probabilities as new information becomes available, allowing for more flexible and accurate predictions. This makes them especially valuable for Ligue 1 predictions where previous match data and trends can influence betting strategies.
How do Bayesian models contribute to data-driven betting strategies?
Bayesian models quantify the uncertainty of predictions and allow for the adjustment of probabilities as new data is introduced. This dynamic nature supports continuous learning from the data, which aligns with the principles of data-driven betting strategies, facilitating more precise wagering decisions.
What are dynamic betting models and how do they work?
Dynamic betting models are predictive tools that adapt to changes over time, capturing the fluctuations in team performances. They account for variables such as player form, injuries, and tactical shifts, allowing bettors to make predictions that reflect the current state of a team.
Why is it important to consider team performance trends in sports betting?
Team performance trends give insights into a team’s momentum, stability, and improvement or decline. In sports betting, understanding these trends can be the difference between placing a winning or losing bet, as they allow bettors to predict future performances based on past and present data.
How does integrating external data improve Ligue 1 betting predictions?
Integrating external data, such as weather conditions, team morale, and market dynamics, provides a more comprehensive view of factors that could impact the outcome of a match. It helps in developing a more sophisticated model for betting predictions by incorporating variables that are not captured by on-field statistics alone.
Why is performance tracking critical in Ligue 1 betting?
Performance tracking allows bettors to follow teams and players over the course of the season, noting changes in form and effectiveness. This continuous monitoring is crucial for understanding how external factors like injuries or team changes impact Ligue 1 betting outcomes.
- What you need to know about betting on eSports? - August 11, 2024
- Combining 27 Express with Traditional Betting - August 2, 2024
- The impact of WAFCON on Ghana’s local women’s leagues - July 29, 2024